Pay Equity Analysis: The Essential Guide [With a Tutorial]
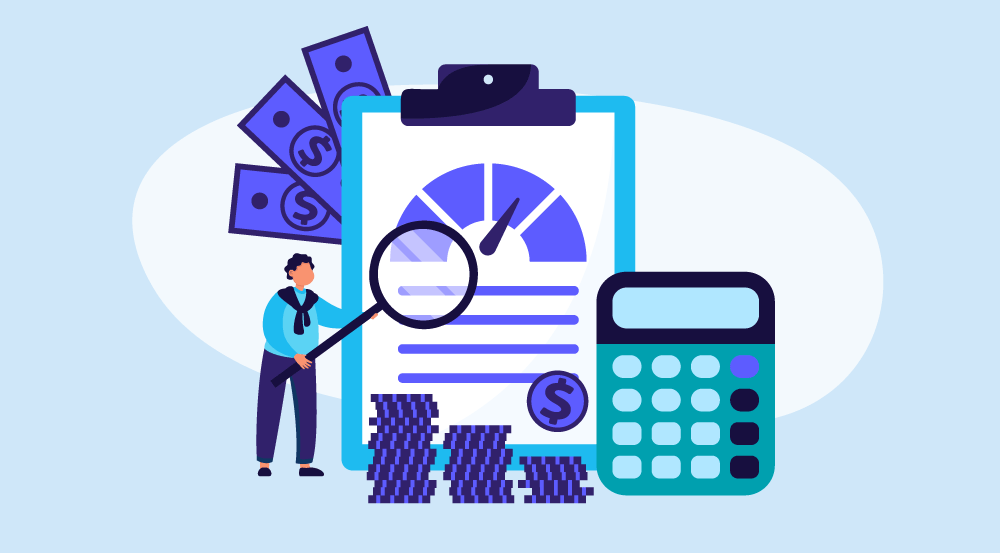
If you’re serious about providing equitable compensation to your employees, a pay equity analysis is an indispensable tool in your arsenal. Let’s look at the pay equity analysis definition, why conduct it, and steps in a comprehensive pay equity analysis.
Contents
What is a pay equity analysis?
Why conduct a pay equity analysis
How to conduct a pay equity analysis
What is a pay equity analysis?
Pay equity centers around the belief that employees should be compensated the same if they’re doing work of equal value. This is defined as work requiring substantially similar skills, responsibilities, and job complexity, performed under similar working conditions.
For example, in a retail store, a male shelf stacker and female sales assistant should, in theory, be paid equally unless there’s a solid reason for a pay difference. A fair difference in pay could be attributed to differences in ability, tenure, and qualifications amongst employees.
HR professionals conduct pay equity analysis (PEA) to understand whether pay disparities exist in an organization. This is done through statistical analysis of payroll data. Employees who perform “like for like” work have their pay compared. Any unjustified differences are noted, with changes proposed to senior management and leadership teams about immediately creating fairer pay structures across the business. A PEA is typically conducted once a year in an organization, but it can be carried out whenever a company sees fit.
Why conduct a pay equity analysis
Many organizations continue to pay women and ethnic minorities less than White men for doing the same work. However, a Glassdoor survey reported that 67% of US employees would not apply for a job at an organization where they believe a gender pay gap exists. Not only are more employees demanding fair pay, but the law is slowly catching up and requires businesses to play ball.
Conducting a pay equity analysis is fair and ethical. It demonstrates your commitment to diversity, equity, inclusion and belonging (DEIB), improves your compensation & benefits structures, helps you stay competitive as an employer in your industry, meet shareholder expectations, and ensure legal compliance.
It’s the responsibility of HR to ensure the organization complies with any relevant pay equity legislation. Failure to do so can result in lawsuits and other legal action, plus it can irreparably damage the organization’s reputation with both employees and customers. Such damage could be more costly than any fines the company faces.
Legal requirements vary across countries and states. In the US, the Equal Pay Act of 1963 states that organizations must pay equal wages for equal work, which means that all organizations based in the US must comply. Other laws, such as the Americans with Disabilities Act (ADA), don’t apply to smaller-scale businesses, so HR leaders must check which legislation applies to their organization.
In March 2021, California passed a new law that requires employers to file annual equal pay reports. Meanwhile, Colorado and multiple other states have passed or are considering passing pay transparency bills. In the New Jersey Diane B. Allen Pay Equity Act, there are 13 protected classes, including gender, race, sexual orientation, and age. In Ontario, the Pay Equity Act requires employers to categorize their jobs as either female-job class (if 70% of employees are female), male-job class (if 60% of employees are male), or gender-neutral (if the number of employees is about the same). These job classes are then compared with ones of equal value, and pay rates must be aligned accordingly.
In Europe, The European Union (EU) monitors and supports the implementation of the Directive 2006/54/EC on equal pay across its member states. What’s more, the European Commission is proposing a new directive on pay transparency, which is meant to reinforce equal pay for work of equal value.
How to conduct a pay equity analysis
1. Set your goals and get buy-in
What is the primary goal of your pay equity analysis?
To ensure you’re eliminating legal risks? To update your current pay practices and policies? To respond to shareholder demand? To eradicate pay inequality amongst employees? Or something else? It’s essential to clarify your goals at the start, as these will shape the process and methodology.
It’s equally important to get leadership buy-in before beginning your analysis. Knowing your ultimate goal will enable you to explain to senior management the purpose of the audit and how it will benefit the organization in the long term. Pay equity analysis requires people, time, and money, so you’ll need to ensure you have the budget and capacity to handle the task. It’s common to require assistance from HR personnel, finance or payroll personnel, and legal counsel to assist with the audit. Additionally, you may want to enlist the help of your analytics team or a data scientist—anyone experienced with regression analysis and statistical software.
2. Analyze your current pay policies and practices
The second step is to take stock of the current policies you have in place. What does your compensation and benefits package look like? If your organization spans across locations, what are the differences, if any? Your compensation and benefits team will likely have a strong idea of where any pay discrepancies exist, so you may want to begin here.
Pay equity analysis is a complex process that can be never-ending. Begin with the basics, for example, deciding whether your current pay policies are fair based on gender and ethnicity, and build from there. For instance, Google was concerned that their employees in customer-facing roles had an unfair advantage that made them more likely to be promoted to higher-paying positions. They conducted a pay fairness audit and found this was not the case.
According to federal law, a difference in pay is justified if an organization can demonstrate the difference is based on one of the following:
- a seniority system
- a merit system
- a system which measures earnings or quantity or quality of production
- any other factor other than sex (also known as the “catch-all” exception, which includes factors like education, training, or shift differential)
However, it’s important to note that the fourth factor has come under scrutiny recently, and many states now require the factor other than sex to be job-related or based on business necessity.
3. Determine what “comparable work” means at your organization
“Comparable work” or “substantially similar work” is typically defined by state law as work that requires substantially similar skills, responsibilities, and input and is also performed under similar working conditions. To determine whether two jobs are comparable, it’s necessary to analyze the job as a whole. This process is called job evaluation. Looking at job titles and job descriptions alone cannot determine compatibility. In addition, you shouldn’t automatically assume that positions in different departments or units are not comparable.
To ensure your organization meets legal requirements regarding pay equity, identifying all employees who perform comparable work is a key step in the process. As there’s an emerging trend for pay equity laws to extend beyond gender, it’s important to check the details of the legislation in your location and identify all comparator groups.
4. Gather the relevant data
The more employees you have, the more reliable your estimates and results will be. We find that results become robust if 250 employees or more are analyzed.
The next step is to begin gathering your data for analysis. You can usually extract most of this information from your HRIS or payroll databases.
Here are some of the variables you could include:
Salary information:
- Base salary
- Bonuses
- Variable compensation
Position information:
- Job title
- Job level
- Skill pool
- Team
- Department
Employee / Contract information:
- Gender
- (Weekly) Working hours
- Age
- Ethnicity
- Tenure
- Seniority
- Sexual orientation
- Performance
- Potential
The more variables you have, the more accurate you can estimate any potential biases. Ultimately, your data file could look something like our example dataset, which you can download here:
jobtitle department salary gender age tenure performance joblevel contract education Software Designer B2B 39621.75 F 58 10+ 4 Consultant 60% PhD Graphic Analyst B2B 20962.63 F 56 <5 3 Consultant 60% Master’s Business Developer Management 73637.43 M 64 5-10 2 Engineer 100% Bachelor’s Marketing Analyst Operations 95765.07 M 42 5-10 3 Director 100% Bachelor’s Software Associate B2B 10617.87 F 31 <5 4 Consultant 20% Master’s Marketing Designer Finance 51247.47 M 35 10+ 3 Analyst 60% Bachelor’s
While gathering data, it’s vital to consider employee privacy. Have a plan in place before you begin your analysis that protects the confidentiality of all your employees. No information should be transferred to your analyst that could personally identify an employee. Remove all sensitive information from your data file before you proceed.
5. Analyze and identify pay differences within your company
Many organizations benefit from inviting their data analytics team or enlisting the help of external experts to assist with this step.
However, it might also benefit you and your HR team to upgrade your People Analytics skills to be able to follow the analysis process. You could enroll in a People Analytics course to improve your data-driven decision-making in HR.
Also, if you’re not a Compensation & Benefits specialist, you might find a Compensation & Benefits course for HR professionals useful to better understand various aspects of pay within organizations.
Now, let’s dive into the analysis process.
i. Install your software
You could choose to use:
- R
- Python
- SAS
- Excel
We work with R in RStudio, both of which you can download for free by following the links.
ii. Data loading & setup
Once you have your data, the next step is to set up your R environment and load the data into R. For this analysis, you’ll need several R packages, which are prewritten code modules.
# install.packages('tidyverse')
# install.packages('broom')
# install.packages(‘kableExtra’)
First, if needed, install the packages locally on your computer. Next, you can attach its functions to your R working environment:
library(tidyverse)
library(broom)
library(kableExtra)
By setting this minimal theme as your default design, your plots will look more aesthetically pleasing:
theme_set(new = theme_minimal())
And you might want to turn off scientific notation:
options(scipen = 999)
Once you have all the necessary packages loaded, you can upload your company data.
Point the file path in the code below to the download location on your local computer.
# We read in the data and store it in memory under the object name `df`
# Be sure to change the filepath to the directory location where the dataset is stored on your own computer
df = read.csv(file = 'data/HRIS-data.csv')
iii. Data cleaning, exploration & preparation
It’s important to leave yourself enough time to familiarize yourself with the data and prepare it for analysis.
We’ve grouped data cleaning, exploration, and preparation in one stage here, as one often leads to another.
For instance, you will need to explore your data before knowing what to clean. Often, during cleaning, you already prepare and set your data up for analysis. And while cleaning the data, you might uncover some new data categories relevant to your analysis.
You need to consider a few things when performing a pay equity analysis with your own organizational data.
Fully understand your salary data
For a pay audit, you want to ensure you understand and isolate the different salary components. These include:
- Base salary
- Performance bonuses
- Stock options
- Commissions
- Special recognition awards
- Claim reimbursements
- Paid sick days
- and many more.
Some of these components are more important and relevant than others, and some might be more prone to display bias than others. It’s sensible to focus your analysis on one salary component at a time. For example, let’s say your base salaries are equally distributed, but your stock options are not. You might not spot this smaller bias in your stock options when looking at the total remuneration package.
When it comes to data cleaning, you might need to remove the financial symbols (e.g., $, €) included in your salary data. In R, you can use the readr::parse_number() function for this.
You may also want to perform some general checks here.
- Is all your salary data positive and above zero?
- Is the salary data within the range of what you would expect?
- How about when you look at hourly rates?
- Is the salary data normally distributed enough, or do you need to apply some transformations before analysis?
Finally, you will want to account for contract hours. An employee who works 20 hours a week will earn less than a full-time employee in the same position. You can account for this either by transforming your salary data to an hourly rate, by extrapolating all salaries to full-time contracts, or by including contract hours in your later analysis as a control variable.
Analyze comparable groups
If you work in a large organization, it might be a good idea to perform a pay audit for multiple entities separately. For example, if you operate in numerous countries, it could be more insightful to repeat the audit for each country’s employees. This ensures that you compare comparable employees and salaries and that no external factors bias your results but remain unaccounted for.
It’s important to note that you need large enough sample sizes for each analysis to ensure robust results from which you can draw sufficient conclusions.
Don’t estimate effects for small groups
One final thing to consider is to avoid including small groups in your analysis. A great example is the use of job titles. You might encounter a wide range of titles in any organization. Some job titles might even be unique to a specific person in an organization. When you have such small groups, a statistical model is often unable to learn and isolate a possible salary effect.
There might be better ways to group such data. For example, it’s often not the job title that determines a salary, but rather the job level for that position. It’s, therefore, better to use that information as input for your analysis.
Similarly, instead of looking at biases in small teams, you might want to look at departments. Instead of city locations, perhaps you could look at regional differences.
If you have many data points you can’t group, consider adding an “other” category.
iv. Explore the unadjusted gender pay gap
Once you’ve cleaned your data and made it relevant, you might want to explore the gender pay gap before jumping into the analysis.
In our data, the salary distributions for men and women look like this:
ggplot2::ggplot(data = df) +
ggplot2::geom_density(mapping = ggplot2::aes(x = salary, fill = gender), alpha = 0.5)
You can clearly see there are relatively more male salaries located to the right of the plot, where the higher salaries reside.
Now, let’s have a look at the unadjusted gender pay gap. This is essentially the difference between the average male and female salaries. We can calculate this unadjusted metric by doing the following:
# Create a boolean index for female data records
is_female = df$gender == 'F'
# Calculate average salary of female data records
female_average_salary = mean(df$salary[is_female])
print(female_average_salary)
## [1] 44106.55
# Calculate average salary of male data records
male_average_salary = mean(df$salary[!is_female])
print(male_average_salary)
## [1] 64290.64
# Calculate unadjusted pay gap by substracting one from the other
unadjusted_pay_gap = male_average_salary - female_average_salary
print(unadjusted_pay_gap)
## [1] 20184.09
This shows that women earn $20,184.09 less than men in our organization on average.
However, this metric is unadjusted for various factors that are known to affect salary, including job level, tenure, previous work experience, and more. Therefore, looking at unadjusted pay gaps is often not as informative as you’d like it to be.
Fortunately, we can use statistical modeling to control all these other salary drivers and isolate the effect of gender. This is what we call the adjusted pay gap.
v. Estimate the adjusted pay gap
We can estimate the adjusted gender pay gap by deconstructing salaries as an equation.
Here’s an equation for the pay of a typical worker:
Salary = Male * B1 + X * Bx + e
Here, the B1 coefficient will reflect the effect that “being male” has on your salary. The impact of all other variables (X) is estimated in their respective coefficients (Bx).
You won’t have information on everything, which is why there will be some random noise in the data. Any unaccounted differences will be captured by e – the error.
Statistical models
Next, we’ll explore running four consecutive regression models. The models become increasingly elaborate, modeling salary as a function of more and more predictors.
Model 1: Salary as a function of gender
The first model is fairly naive because it assumes that only gender affects your employees’ salary.
mod1 = lm(salary ~ gender, data = df)
With our data, this model shows that male employees earn on average $20,184.09 more than their female colleagues.
This effect is highly significant, as shown by the p.value in the last column (approximately 0.000).
broom::tidy(mod1) %>%
kableExtra::kbl(caption = 'Model 1', digits=2) %>%
kableExtra::kable_styling()
Model 1
term estimate std.error statistic p.value (Intercept) 44106.55 852.54 51.74 0 genderM 20184.09 1298.63 15.54 0
Model 2: Considering the implications of working hours
The second model considers a slightly less naive scenario by adding the employees’ contract hours information to the salary equation.
We know these to have a very strong effect on annual salaries, and the percentage of full-time employees might not be equal among men and women.
mod2 = lm(salary ~ gender + contract, data = df)
With our data, the results of this model show that contract hours account for a large chunk of an employees’ salary. Also, the gender bias found in our first model has shrunk considerably.
broom::tidy(mod2) %>%
kableExtra::kbl(caption = 'Model 2', digits=2) %>%
kableExtra::kable_styling()
Model 2
term estimate std.error statistic p.value (Intercept) 62395.32 896.23 69.62 0 genderM 8126.63 965.69 8.42 0 contract20% -49708.75 2087.31 -23.81 0 contract40% -37822.90 1552.52 -24.36 0 contract60% -25493.94 1224.82 -20.81 0 contract80% -9736.66 1217.52 -8.00 0
Our data indeed showed a large difference in the percentage of male vs. female full-timers:
df %>%
dplyr::count(contract, gender) %>%
tidyr::pivot_wider(names_from = gender, values_from = n, values_fill = 0) %>%
dplyr::mutate(pct_women = F/(M+F)) %>%
dplyr::arrange(pct_women) %>%
kableExtra::kbl(caption = 'Gender x Fulltime Contracts', digits=2) %>%
kableExtra::kable_styling()
Gender x Full-time Contracts
contract F M pct-women 100% 140 272 0.34 60% 122 47 0.72 80% 130 45 0.74 40% 69 20 0.78 20% 46 0 1.00
Yet, this difference in contract hours does not eliminate all salary bias. This second model still shows that male employees earn on average $8126.63 more than their female colleagues.
Again, this effect is highly significant as shown by the p.value in the last column (approximately 0.000).
Alternative approach
An alternative approach to account for working hours would have been to define a different outcome variable. For example, you could have calculated something like salary_per_hour_worked, by simply dividing the salary by the contract hours or the part-time percentage. If you used that newly calculated variable as your dependent variable in your regression equation, you would not have to control for contract in your model.
For illustrative purposes, we stuck to base salary in our models. This allows us to interpret all other effects with more ease.
Model 3: Adding more employee characteristics
We’re adding other employee characteristics to the salary equation in our third model.
mod3 = lm(salary ~ gender + contract + education + age + tenure, data = df)
Our results show that these characteristics account for another large chunk of the employees’ salary variations. As a result, the effect of gender shrinks even further.
However, some bias remains in this third model. Male employees are shown to earn an average of $4309.35 more than their female colleagues per annum.
Again, this effect is highly significant, as shown by the p.value in the last column (approximately 0.000).
broom::tidy(mod3) %>%
kableExtra::kbl(caption = 'Model 3', digits=2) %>%
kableExtra::kable_styling()
Model 3
term estimate std.error statistic p.value (Intercept) 53013.00 1595.36 33.23 0.00 genderM 4309.35 878.65 4.90 0.00 contract20% -51740.47 1831.03 -28.26 0.00 contract40% -38947.10 1355.56 -28.73 0.00 contract60% -26090.94 1069.81 -24.39 0.00 contract80% -10826.54 1063.19 -10.18 0.00 educationMasters 1293.31 839.73 1.54 0.12 educationOther -2123.80 1318.06 -1.61 0.11 educationPhD 5035.46 1303.38 3.86 0.00 age 78.97 27.85 2.84 0.00 tenure10+ 14416.96 939.59 15.34 0.00 tenure5-10 9520.11 915.78 10.40 0.00
Model 4: Considering all available HR information
Our fourth model includes all the remaining human resource information in the salary equation. We add the variables for performance, job level, and department, and for each one, our linear model will try to estimate its effect on salary.
mod4 = lm(salary ~ gender + contract + education + age + tenure + performance + joblevel + department, data = df)
Looking at the results, we see that gender bias has finally disappeared. While the average salary among comparable employees is still $647.37 higher for men, this difference is no longer significant, as shown by the p.value in the last column (approximately 0.377).
broom::tidy(mod4) %>%
kableExtra::kbl(caption = 'Model 4', digits=2) %>%
kableExtra::kable_styling()
Model 4
term estimate std.error statistic p.value (Intercept) 48602.65 1781.18 27.29 0 genderM 647.37 732.79 0.88 0.38 contract20% -51446.69 1440.49 -35.71 0 contract40% -38742.67 1070.72 -36.18 0 contract60% -26044.01 845.53 -30.8 0 contract80% -11753.77 835.48 -14.07 0 educationMasters 141.09 668.28 0.21 0.83 educationOther -3756.89 1041.48 -3.61 0 educationPhD 4437.42 1027.69 4.32 0 age 103.89 21.93 4.74 0 tenure10+ 15372.98 741.96 20.72 0 tenure5-10 9647.82 721.19 13.38 0 performance 7.5 250.32 0.03 0.98 joblevelAssociate 492.57 949.32 0.52 0.6 joblevelConsultant 434.31 943.49 0.46 0.65 joblevelDirector 22128.82 1209.82 18.29 0 joblevelEngineer 3856.74 1077.2 3.58 0 joblevelLead 2901.21 1157.75 2.51 0.01 joblevelManager 6456.59 1421.24 4.54 0 departmentB2C 958.16 1256.76 0.76 0.45 departmentFinance 2121.62 1255.24 1.69 0.09 departmentHR -475.85 1246.73 -0.38 0.7 departmentManagement 9420.99 1174.28 8.02 0 departmentOperations 1203.89 1175.71 1.02 0.31 departmentOther -1062.46 1235.79 -0.86 0.39 departmentSales 942.85 1142.06 0.83 0.41
Of the variables entered in this last step, particularly joblevel shows a strong relation to salary.
A director would earn $22,128.82 more than the referent category of analysts, while the relative salary increase of a team lead is only $2,901.21.
If relatively many men occupy director positions, this could have led to the strong gender pay gap we witnessed in model 1.
Some quick retrospective analysis shows that this is indeed the case.
We see two patterns that amplified the original, unadjusted pay gap:
- Between gender categories, we see men are overrepresented in the higher-salaried positions
- Within gender categories, we see a larger proportion of men in the higher-salaried positions
df %>%
dplyr::group_by(joblevel) %>%
dplyr::summarize(
n = n(),
women = sum(gender == 'F'),
men = sum(gender == 'M'),
average_salary = mean(salary)
) %>%
dplyr::ungroup() %>%
dplyr::mutate(
pct_men = format_pct(men/n),
pct_of_men = format_pct(men/sum(men)),
pct_of_women = format_pct(women/sum(women))
) %>%
dplyr::arrange(desc(average_salary)) %>%
kableExtra::kbl(caption = 'Job level x Gender', digits=2) %>%
kableExtra::kable_styling()
Job level x Gender
joblevel n women men average_salary pct_men pct_of_men pct_of_women Director 84 26 58 76819.79 69.00% 15.10% 5.10% Engineer 132 32 100 58925.89 75.80% 26.00% 6.30% Manager 51 26 25 53269.4 49.00% 6.50% 5.10% Lead 90 56 34 51815.82 37.80% 8.90% 11.00% Associate 179 115 64 48608.76 35.80% 16.70% 22.70% Analyst 165 121 44 47460.75 26.70% 11.50% 23.90% Consultant 190 131 59 46875.75 31.10% 15.40% 25.80%
6. Interpret the data
The next step is to interpret the data. Through the four linear regression models, you can formulate increasingly complex equations to estimate salary. These equations allow us to isolate any linear differences between gender groups.
Although the most simple and naive model displayed an unadjusted gender pay gap of $20,184.09, the final model that took into account all the HR information we had available showed that the adjusted gender pay gap is only $647.37 and was not statistically significant.
Job level and the tenure of employees (amongst other factors) had very strong salary implications. Meanwhile, smaller salary effects can be attributed to employees’ educational level, age, and performance evaluation of last year.
With some certainty, this pay equity analysis allows us to conclude that the observable, unadjusted gender pay gap is not directly related to gender and is caused by other influencing factors. Fortunately, the adjusted pay gap in our final results suggests that we are paying our talent fairly and equally for equal work.
Further research
We can now interpret, explain, and understand what causes the difference in pay in our organization; however, it still exists. Significant differences were visible in the unadjusted measure, which hints at other underlying problems.
Although the models show we are likely paying equally for equal work, there may be other issues at play. For example, we might not be hiring equally. Or we might not promote women as often or as quickly as their male colleagues. There is also a possibility we might not sufficiently assist women in balancing their work and home lives, which leads to them dropping out of the workforce early due to competing roles and responsibilities.
Regardless of the statistical results of your pay equity audit, you should always be thinking of ways to improve diversity, equality, inclusion, and belonging in your organization.
Nonlinear effects
Not all factors that influence salary might have a linear effect. For example, while your C&B policy may cause the salaries of new hires to grow rapidly, employees might reach their salary-bound cap when they have longer tenure. This could cause the effect of tenure to be nonlinear, whereas, in our model, we assumed it was linear.
More importantly, the gender bias in our organization may not be linear and similar. For example, we might not be biased in the way we allocate salaries in the lower levels of the organization, but we might be among Directors. Or there might not be a gender bias in the HR organization, but there may be one in the Sales organization.
You could expand on this by modeling such contextual biases in your organization. For example, by running a pay gap analysis for specific subgroups like job levels or departments, or by adding interaction terms to your general analysis.
Other salary components
Our example only analyzed the gender bias present in base salaries, but you might want to investigate other components.
7. Share the results with key stakeholders and take action
The final step is to take the results from your pay equity analysis report and communicate them with the leadership team and stakeholders, even if they’re not what you hoped for. It’s equally important to let everyone in the organization know that you take pay equity seriously and take the necessary steps to find and address any imbalances. If you’ve identified pay gaps that are not justified by law, it’s essential to correct these as soon as possible.
Over to you
Pay equity analysis is a complex process; however, it is essential to staying compliant with the laws and building an inclusive, equitable workplace. Remember, even if your results are not-so-favorable, they are a great start to ensuring pay equity in the future.
Weekly update
Stay up-to-date with the latest news, trends, and resources in HR
Learn more
Related articles
Are you ready for the future of HR?
Learn modern and relevant HR skills, online